Meet LALAL.AI Cassiopeia: A Milestone in Music Source Separation
Our new audio-track splitting network was trained, tested, and proven to be superior to the leading audio separation solutions on the market. Learn all about Cassiopeia, check out the quality test process and results, and try it yourself!
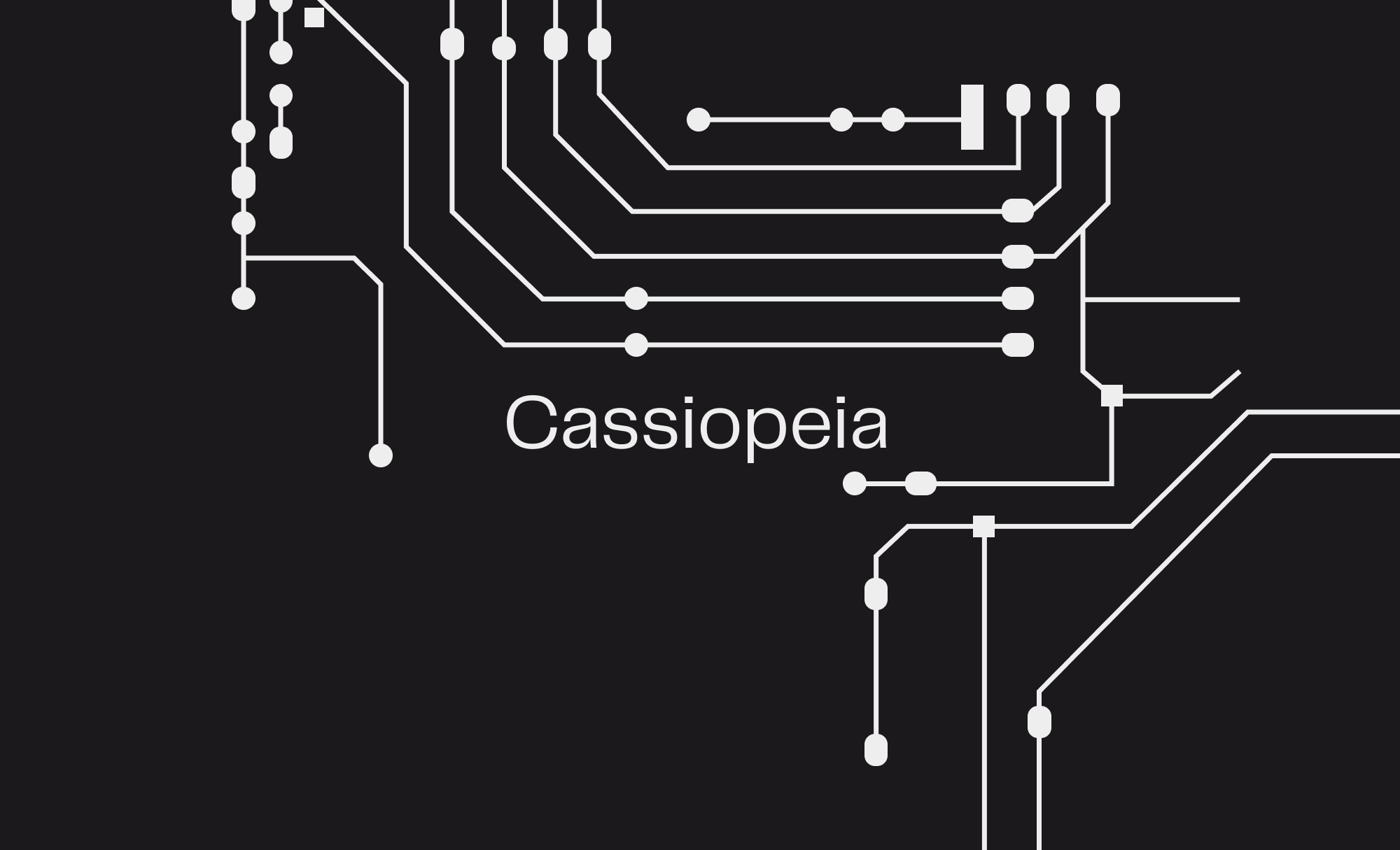
LALAL.AI starts the new year of 2021 with a leap into the future of stem splitting. We are happy to present Cassiopeia, a new source separation neural network we’ve recently trained and implemented.
It’s a next-generation solution relative to Rocknet, the original LALAL.AI neural network, but with new architecture and more advanced capabilities. All of that makes for improved splitting results with significantly fewer audio artifacts and unnatural sounds.
Both Rocknet and Cassiopeia are available for trial and use on the LALAL.AI site. It may take longer for the new network to produce results, however, they can be more precise and clean than those of Rocknet.
Below you can learn more about Cassiopeia, what makes it different from Rocknet, and how it compares to other popular music source separation solutions.
What's New in Cassiopeia
One might assume that Cassiopeia is a successor to Rocknet but it’s actually a completely new neural network architecture. Comparable to Rocknet in complexity, Cassiopeia’s breakthrough capabilities of tracking the input and output signal phase components are unparalleled.
Both solutions work in the frequency domain but Rocknet only considers the amplitude component while ignoring the phase component. This can lead to various unpleasant effects that give separated stems a certain dry, plastic sound.
Cassiopeia, on the other hand, contains an advanced accounting mechanism for the phase component of the input signal and generates the phase for the output signal. Because of that, it’s possible to get rid of a significant portion of audio artifacts.
Test Objective
The rise of AI technologies is having a significant impact on progress in the audio source separation(1) area. We want to test and compare the splitting results of the leading open-source stem separation programs on the market with the LALAL.AI Rocknet and Cassiopeia editions. Our objective is to prove the superiority of the new Cassiopeia neural network to its rivals.
LALAL.AI Contestants
Due to their popularity and satisfactory performance, the following solutions were selected for the comparison with the Rocknet and Cassiopeia solutions:
- Spleeter (2, n.d.) is a solution by Deezer, a popular French music streaming service. It’s available as a source code (3) for separation and as a neural network model that was trained by AI experts from Deezer.
- OpenUnmix (UMX) (4) is a neural network solution from Yuki Mitsufuji and Stefan Uhlich, music industry luminaries that work in Sony's core divisions.
- Extended Unmix (CrossNet-OpenUnmix, X-UMX) (5), (6) is a next-generation neural network by the same authors.
Comparison methodology
All of the above solutions were used to produce the vocal and instrumental tracks. The solutions that allow you to single out the vocal and instrumental track (Spleeter and LALAL.AI) were used in this mode. The solutions that don’t provide direct extraction of the two stems, such as UMX and X-UMX, were used to extract the vocal track, while the instrumental track was obtained by "subtracting" the vocal track from the original composition.
Obviously, the quality of separation is determined by how well you managed to isolate individual stems from the original stereo content. SDR (Source-to-Distortion Ratio) is used as an integral indicator of separation quality. For each of the selected stems, SDR characterizes how much the signal (in this case the vocal signal for the vocal track and the instrumental signal for the instrumental track) exceeds noise and distortions.
Two kinds of distortions usually occur during music source separation:
1) The content of one track gets into the other track, for example, when part of the vocal signal ends up in a separated instrumental track or vice versa. This kind of distortion can be characterized with SIR, the source-to-interference ratio.
2) The emergence of additional sounds that were not present in the original stereo recording. This kind of distortion can be characterized by the SAR, source-to-artifact ratio.
A description of SDR, SIR, and SAR can be found in this article (8). There are generally recognized implementations of methods for calculating these metrics, which can be found in the repository (9). We will also use the latter to evaluate the quality of the solutions.
Testing process
The solution testing process includes several stages:
- Selection of the material to be tested, the so-called test set.
- Separation of each composition from the test set using all of the solutions.
- Calculation of SDR, SIR, SAR for each composition and solution.
- Averaging metrics across compositions for each of the solutions
For the test, we randomly selected six songs from different genre categories:
- 'S Wonderful by Diana Krall (jazz)
- Don't You Remember by Adele (soft rock)
- The Voice by Celtic Woman (Celtic)
- Celui qui reste by Sébastien El Chato (pop)
- A View To A Kill by Duran Duran (synthpop)
- Swing Supreme by Robbie Williams (jazz)
All compositions were separated using all the separation solutions mentioned. The default settings were used with the exception of the following:
- For Spleeter testing, we used the Spleeter-16KHz model was used because the default model has frequency range limitations, which would be unfair to compare with.
- For UMX, the UMX-HQ model was used, which is trained on the uncompressed MUSDB-HQ content. This one delivers better quality in comparison to the default UMX.
The values were then averaged over all compositions. A visualization of the final result is depicted on the graph below.
Test results
Cassiopeia clearly outperforms all its rivals in terms of the overall quality of the instrumental part extraction, characterized by the SDR value. Even more significant is the gap in the instrumental SIR, which can be interpreted as significantly less percolation of the vocal channel into the instrumental one. Spleeter wins by the artifact generation parameter, which is reflected in the SAR metric.
In the vocal channel, Rocknet is still the champion in all three metrics. Cassiopeia has more infiltration of the instrumental channel into the vocal one. This is why there is such a difference in SIR between Cassiopeia and Rocknet, and the same fact is reflected in the difference in SDR.
However, it should be noted that even though SDR/SAR/SIR metrics are objective criteria of separation quality, they don't always adequately reflect the subjective, audible quality. And the case of the vocal channel demonstrates this perfectly.
Although Cassiopeia lags behind Rocknet in terms of formal metrics for vocals, both the instrumental part and especially the vocal stem separated by Cassiopeia sound much more natural and softer than Rocknet's, without the metallic-sounding artifacts that are so characteristic of the other solutions.