How LALAL.AI Works and How to Improve Its Splitting Results
Learn why we went the AI-route, how our neural network was trained, and what you as a user can do to ensure precise vocal & instrumental tracks extraction.
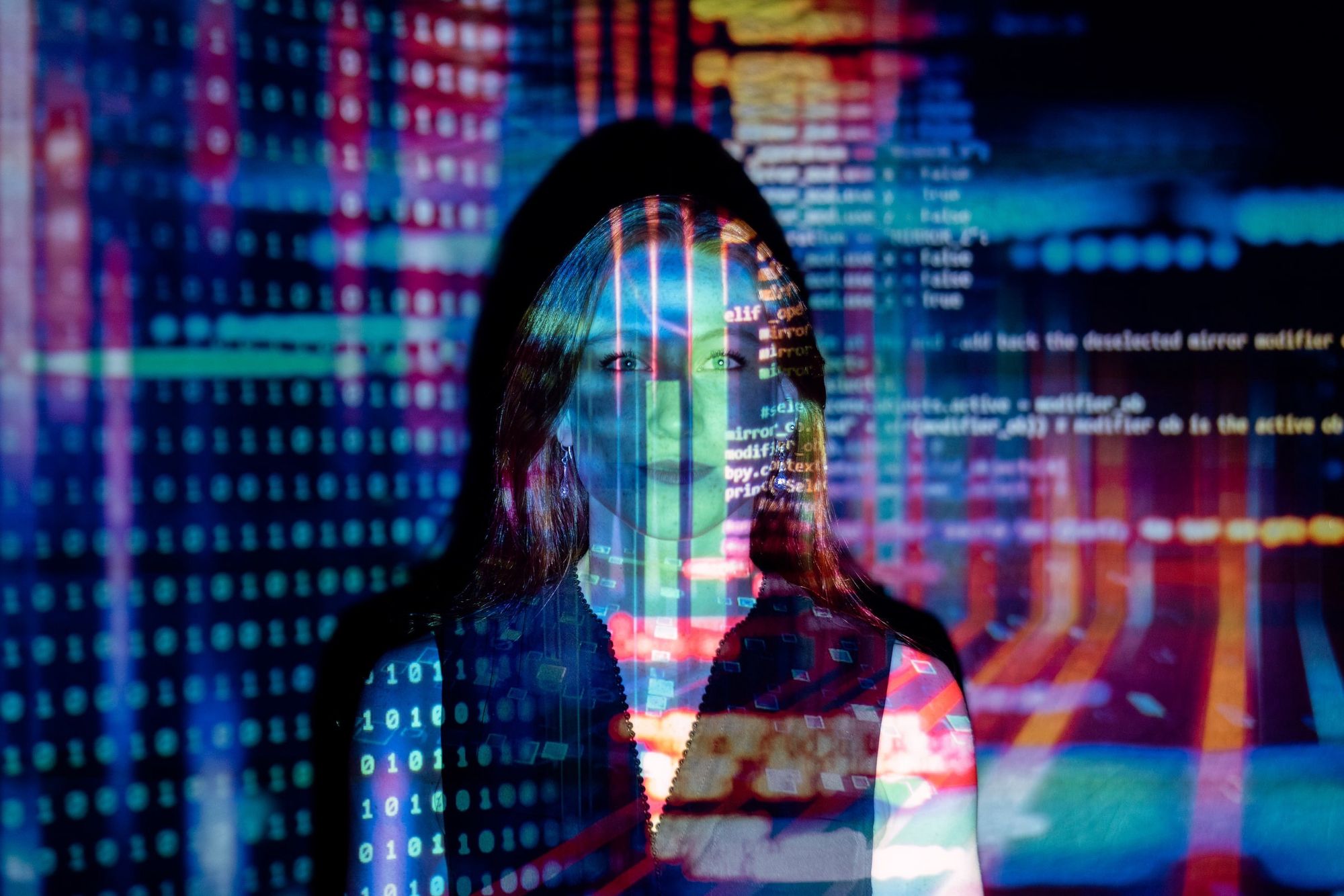
Despite the fact that many songs uploaded to our service are separated into vocal and instrumental tracks with remarkable accuracy, this isn’t the case for all audio tracks. As we mentioned in the LALAL.AI vs. Spleeter comparison post, stem separation precision doesn’t fully depend on the work of these or any other similar services. The quality of sound and mastering of each individual track is the critical factor in how accurate the split is going to be.
As the number of LALAL.AI users grows rapidly, so does the amount of requests to improve the stem separation result of this or that song. As much as we would like to, we have no direct influence on the splitting quality of specific audio tracks. Vocal and instrumental stems are extracted by a neural network, not by a manual human input.
How Artificial (and Human) Intelligence is Trained
The acquisition of intelligence, whether artificial or human, is not some magical act of instant enlightenment but a process of learning. Modelling is an essential part of this process. When we imagine how a particular thing works, we build models using its relevant aspects and properties and set expectations about its behavior. The more accurately the model reflects the modeled object’s behavior, the better the model is.
Learning is an iterative process that involves influencing the object and the model in the same way, comparing the responses obtained from the model and the object, adjusting the responses to the closest (ideally, complete) match, and repeating this procedure until the model’s responses are good enough for every possible input. Essentially, learning is about optimizing created models. In the case of machine learning, the model optimization is executed with the help of a computer.
The Difference Between Regular and AI/ML-Powered Services
Creating algorithms (optimized models) from data is the most important aspect of machine learning. In contrast to systems that follow strict rules and perform tasks in the same way every time, machine learning algorithms improve with experience by learning from more input data.
Computers are modelling different things, processes, and phenomena all the time. For example, any text editing software is a model of a typewriter, a digital calendar is a model of a paper calendar, Excel is a model of a checkered notebook. Although these models are significantly more advanced than their objects (real-life equivalents), they cannot be considered ‘intelligent’ since they do not learn and only repeat pre-programmed behavior.
Similarly, the stem separation quality provided by DAW plugins or any conventional software won’t be improving as you add more data, while the results of the LALAL.AI stem splitting is going to get better over time because of the machine learning algorithms.
How the Artificial Intelligence of LALAL.AI Works
As it was previously established, AI creation requires the following:
- A proper model (also known as a “model” in AI/ML).
- A proper model optimization process (called “training” or “learning” in AI/ML domain).
LALAL.AI has both. The model we use is unique and very complex. The neural network processes stereo sound from various input audio formats, then transforms it into two stems. The network generates a data fragment describing the placement of vocal and instrumental parts in the original input signal. After that, the data fragment is passed over to another, much simpler algorithm that converts the input signal into separate vocal and accompaniment stems.
Seems trivial? It is! As soon as a neural network is designed, applying it is easy… If that’s the word you would use for performing several billion math operations. The network training process is quite a challenge, though.
Just like a small child that needs to do something several times before learning how to do it correctly and without adult help, the neural network needs to process a hundred or even thousands of audio tracks to learn how to perform stem separation properly. Thousands of songs could easily equal dozens of gigabytes of training data.
Splitting just a single second of an audio track takes hundreds of millions of mathematical operations. Considering that an average song is about three minutes long, separating its vocal and instrumental tracks induces billions of math operations. Correcting mistakes and improving the splitting model multiplies those billions by a hundred resulting in nearly a quadrillion operations! Imagine how much computing resources are needed to design a neural network like the one LALAL.AI operates on.
When it comes to machine learning and artificial intelligence, you have to go big. The amount of data, the required computational power, the time spent on training and optimization — everything is huge. But if you’re ready to invest this amount of resources, you can achieve unbelievable results!
How to Improve LALAL.AI Separation Results
- Adjust the audio processing level. Some songs may require additional polishing due to the containing mastering peculiarities and errors that only become audible after the splitting. Change the level from the default Normal mode to Aggressive and check how the separated stems sound after that.
- Find and upload another version of the song or try another track. Look for the same song but with a higher bitrate or in a lossless format. It’s also possible that there are alternative studio recordings for the track in question, the versions that allow for a more refined stem extraction.
We are also planning to implement a feedback system in the near future. Users will be able to select particular stem parts that were poorly separated. We are sure that this update is going to significantly improve the overall splitting quality, and address the issues that specific audio tracks present.
Stay tuned!