LALAL.AI Presents Phoenix, A New Step in Evolution of Audio Source Separation
Meet Phoenix, our new neural network that isolates stems faster than ever before and provides better vocal separation quality than all other AI-based stem splitters on the market.
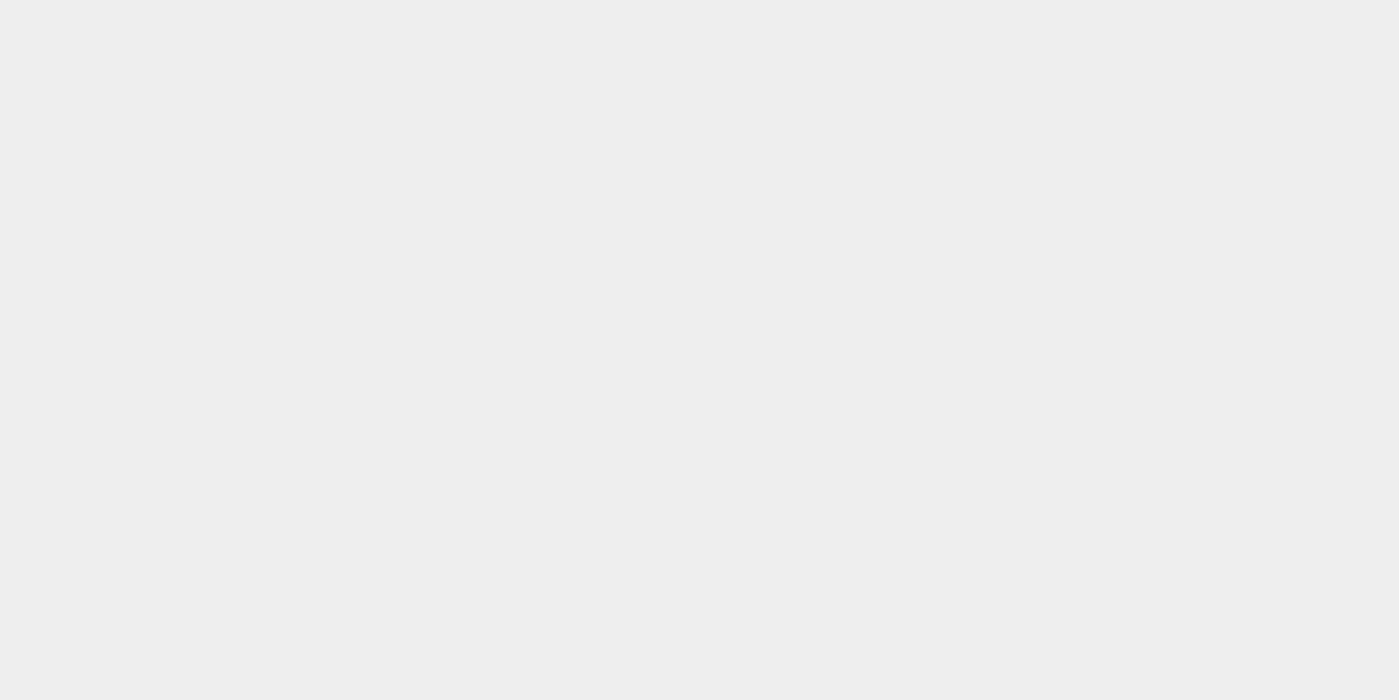
Less than a year ago, we introduced Cassiopeia, an audio source separation solution that surpassed Rocknet, the original LALAL.AI neural network, both in quality and accuracy. Since then, we’ve had tons of new ideas about the ways Cassiopeia's algorithm could be improved, and the development of machine learning and artificial intelligence has not stood still.
In order to bring LALAL.AI closer to our idea of an ideal separator, we did a lot of research and identified the most promising new approaches that became the foundation of the next-generation neural network called Phoenix.
Compared to the previous neural network, Phoenix processes and splits files into stems twice as fast, delivers higher quality vocal extraction, handles backing vocals much more carefully, and produces significantly fewer artifacts.
Below you can find detailed information about the new neural network and how it came to be, evaluate the quality of separation on concrete examples, and learn about our plans on the service development.
A Strive For Better Quality
At the time when LALAL.AI was still using Rocknet, our first-generation neural network, we were analyzing the network performance and studying all existing and popular AI-based stem separators to improve the stem splitting quality of our service. As a result, we came to the conclusion that all solutions, including ours, have the same key flaw - they focus on amplitude processing and ignore the phase aspects. It was a prerequisite for the creation of new architecture, subsequently used for Cassiopeia, LALAL.AI’s second-generation neural network.
It was mentioned in our Cassiopeia article that ignoring the phase aspects leads to artifacts that sound alike in all of the aforementioned solutions. These artifacts produce a dry, plastic sound, and sometimes effects similar to those generated by sound processors. Both are quite unpleasant to the ear and perceived as foreign in isolated stems.
There is a reason why most solutions don’t take phase into account. It’s an extremely sophisticated task due to the specific and instrument-dependent behavior of the phase. Attempts to solve the phase inclusion problem head-on, for instance, by repeating the way amplitude processing is performed, don’t work. It’s demonstrated in open publications on the topic and proven by our own investigations.
Cassiopeia - A Unique Solution
Despite the complexity of the problem, our researchers managed to find the key to it. They created a neural network architecture that could work in the complex number field (all solutions before worked in the field of real numbers) and process both the amplitude and phase parts of the input mix and output stems simultaneously. It was a completely new network structure that had very little in common with Rocknet, the neural network LALAL.AI operated on at the time. The new neural network was called Cassiopeia.
Cassiopeia provided a leap in separation quality, outperforming Rocknet by a whole 1dB of SDR (signal to distortion ratio) in vocal isolation. In addition to the superiority in formal quality, the stems generated by Cassiopeia had a much fuller, denser and more pleasing sound due to the correct phase processing.
However, the higher quality came at a high price. Cassiopeia is a far more complex network than Rocknet. Cassiopeia’s training required almost a terabyte of data and machine time just to isolate the vocal channel - it’s comparable to a year of operation of an average gaming computer.
Phoenix - The New Stage of Evolution
The researchers at LALAL.AI have an idea of the quality that stem separation solutions can have. In spite of the greatly increased separation quality, Cassiopeia still didn’t meet our vision of the perfect stem separator.
During the development of Cassiopeia, we’ve got a lot of ideas on how its algorithm could be improved. We also closely followed the active development of machine learning and artificial intelligence. It seemed that some of the approaches invented in the fields of image and natural language processing could be applied to audio as well, including source separation.
We did a lot of research to determine the best approaches and ideas that formed the basis of the next-generation neural network called Phoenix. The ideas underlying the new neural network can be divided into three groups:
- Input signal processing method.
- Architectural improvements.
- Separation quality evaluation methods.
As the neural network processes an audio file, it divides a track into segments and “observes” each of them one at a time. The main thing in the first group is the increase in the amount of data that the network “observes” at a time to figure out the composition of the instruments and isolate the required ones, such as the voice or the drums. For Cassiopeia, the size of the segment is 1 second, for Phoenix it’s 8 seconds. Because Phoenix “observes” more data, it’s able to recognize the instruments that make up the composition and the characteristics of the sought-for source better.
In terms of theoretically achievable separation quality, the larger the array of observed data, the better. In practice, however, an expansion of the data segment leads to an increase in network complexity, as well as an increase in both the time required for network operation during separation and the time required to train it.
For example, increasing the segment from 1 second to 8 seconds for Cassiopeia would make the network impossible to be trained in a reasonable amount of time, and even when trained, the network would be so slow that users would have to wait dozens of minutes to get separated stems.
Phoenix's architectural improvements alone allowed us to both increase the amount of observed data and cut the network's runtime almost in half! For our users, this means the song processing takes twice as little time. In addition, we’ve improved our server farm, so that the separation time is even shorter.
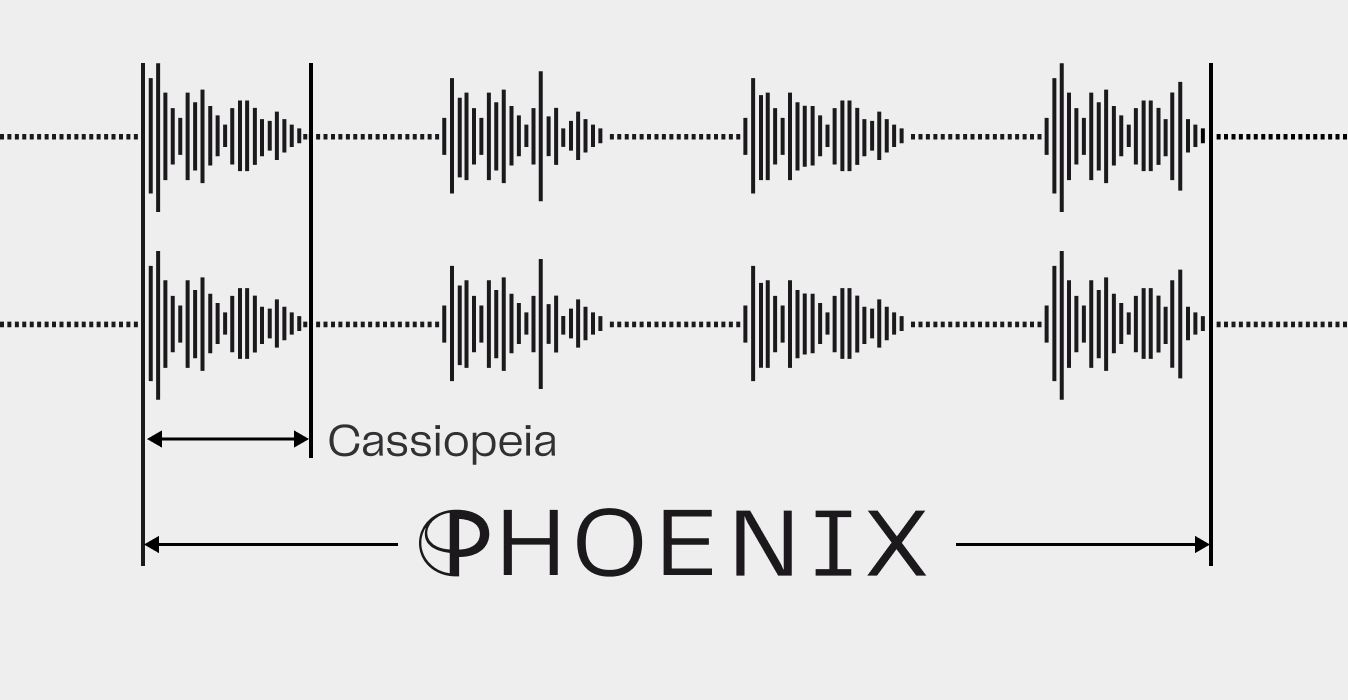
In the second group, there are a lot of different improvements. For example, we borrowed new activation functions for neurons from computer vision and adapted them for audio processing. We applied more advanced methods of normalization which allowed us to balance the network better and make it more trainable.
The third group is probably the most important. In order to evaluate any solution, we need criteria. To evaluate the quality of stem separation, we need criteria of separation quality. Moreover, the criteria are needed during neural network training because the network not only needs to understand when it separates well and when it separates badly but also to understand what it needs to do to separate better.
Researchers need quality criteria to determine whether the resulting solution meets their quality expectations. Unfortunately, the perception of audio is very subjective. It’s quite difficult to find formal mathematical metrics that, when calculated for audio material, would clearly and consistently describe certain aspects of it.
In the case of separation, it’s difficult to make numerical measures that reflect the subjective quality of the resulting stems. More precisely, up to a certain level of quality, it’s easy to construct the measures, they are widely known. There are metrics that enable us to distinguish bad from terrible quality. However, there are no metrics that allow us to differentiate between good and great quality.
We had to investigate in order to establish a mathematical foundation that best represents the quality of separation that ranges from good to excellent. The group of found metrics was used as the basis for training and quality assessment of the new Phoenix neural network.
Phoenix in Figures
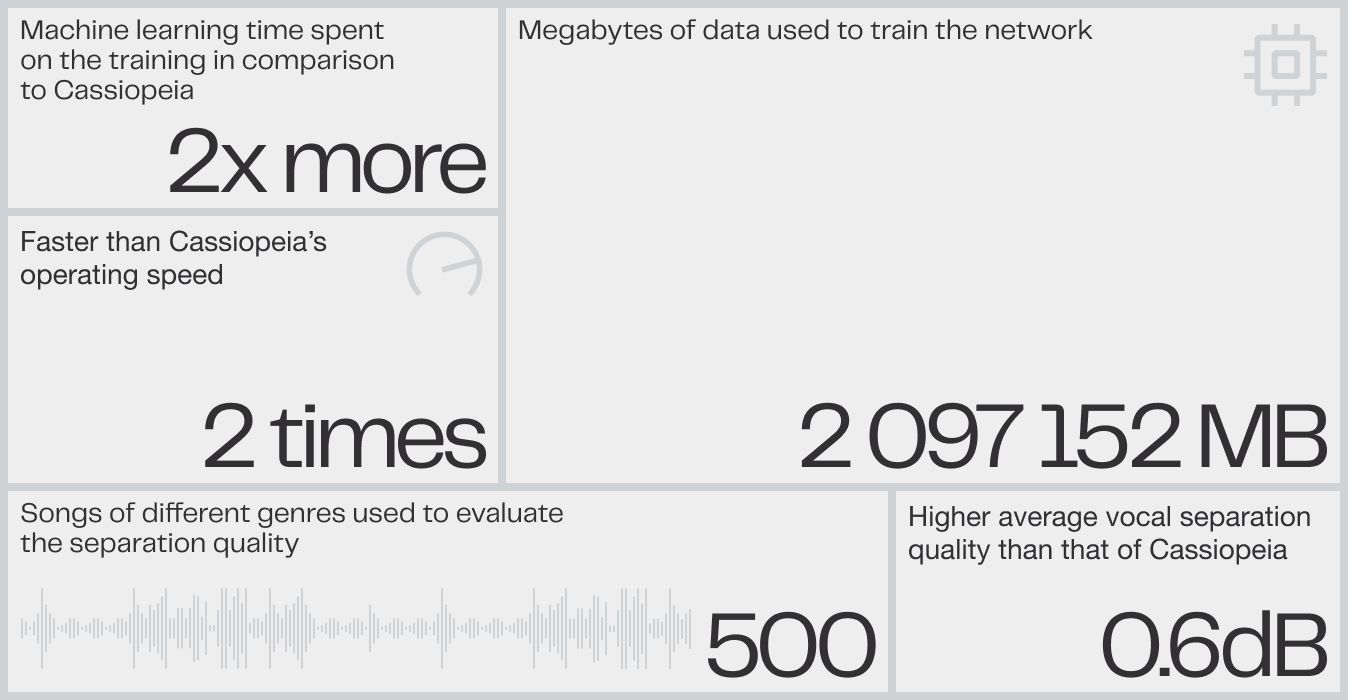
This is what the Phoenix neural network looks like in numbers:
- 2 terabytes of data were approximately used to train the network to extract the vocal stem alone.
- 2x more machine learning time spent on the training in comparison to Cassiopeia (equals to about two years of operation of an average gaming computer).
- 2 times faster than Cassiopeia’s operating speed.
- 0.6dB higher average vocal separation quality than that of Cassiopeia. Just to remind you, the quantum leap in quality from Rocknet to Cassiopeia was 1dB. The gain in quality offered by Phoenix is at least comparable.
On our dataset of 500 songs of different genres that we use to evaluate the separation quality, Phoenix has SDR, a median signal to distortion ratio (a metric that does not fully, but still well enough, reflects the separation quality and is easily explainable) of about 9.5dB, whereas Cassiopeia has 8.9dB.
If you draw a histogram of the quality of separation by test tracks, Phoenix will look as follows in comparison with Cassiopeia:
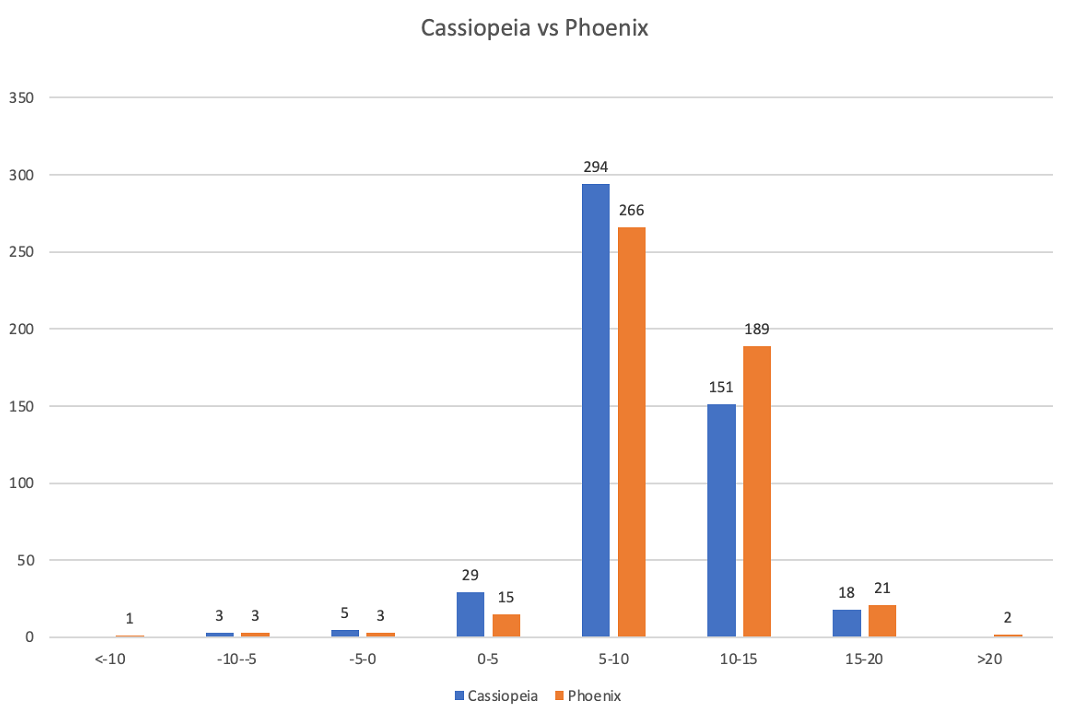
The horizontal marks show the ranges of separation quality in terms of SDR. The smaller the value, the worse the separation quality. So, for example, values less than 0 characterize bad quality of separation, values greater than 15 - excellent, values 5-10 - conditionally average, 10-15 - conditionally good.
We can see that Phoenix separated almost a quarter more songs with good quality (189 vs. 151) and correspondingly nearly as many fewer songs with average and bad quality.
Examples
In terms of the subjective perception of Phoenix results, we can distinguish two general trends:
- Significantly more accurate work with the backing vocals, especially those that sound in unison with the main vocals.
- Significantly fewer artifacts in the vocal channel that could be characterized as dry, “sandy” sound.
A few typical examples of Cassiopeia and Phoenix are given below.
Disclaimer: The following materials are for informational purposes only.
Work with backing vocals:
In this fragment, you can hear that in the stem produced by Phoenix, the backing vocals are clearly defined while in the results of Cassiopeia the backing vocals are lost.
In the fragments below you can hear how the Cassiopeia results contain artifacts in the form of background noise that can be described as “sandy” sound, while Phoenix does not produce such defects. You can hear the difference even on consumer audio equipment but studio-level headphones or monitors will do much better for this task.
Phoenix also does a much more delicate job not only with the backing vocals but also with the main vocal part:
In addition, Phoenix tends to allow much less non-vocal seepage into the vocal stems. You can hear it in the examples below:
All of the above is also true for the instrumental channel. However, it’s much harder to hear the difference due to the much more complex structure of the instrumental part, as well as the psychoacoustic specifics of audio perception, especially without the appropriate studio-grade equipment.
Further Development
At the moment we have a solution for isolating the vocals of the Phoenix class. There is no doubt that it’s going to be followed by solutions for separating other stems - bass, drums, piano, electric and acoustic guitars, synthesizer. It's only a matter of time since each of these requires a substantial amount of machine time to create.
Follow LALAL.AI on Twitter, TikTok, Instagram, Facebook, YouTube, and Reddit to be up to date with all news about the service.